A Guide to Data Transformation Concepts and Implementation
Uncover modern data transformation techniques and explore Prophecy's tools for seamless implementation. Elevate business insights through effective data management.
Whether you're preparing datasets for analysis, integrating data from multiple sources, or building machine learning models, data transformation remains an essential step in the process. But the explosive growth of data volumes and increasingly complex modern data sources has fundamentally changed how organizations tackle this critical function.
The data transformation landscape today bears little resemblance to what existed just a few years ago. Businesses now manage petabytes instead of gigabytes, work with streaming sources rather than batch uploads, and wrangle unstructured formats instead of neat tables.Â
This shift has sparked innovative approaches to data transformation that prioritize scalability, automation, and real-time processing.
This article explores the core concepts of modern data transformation, traces the evolution from traditional ETL to newer paradigms, and offers practical guidance on implementing effective transformation workflows in today's complex data environment.
What is data transformation?
Data transformation is the process of converting data from one format, structure, or value to another. This critical function in data management involves manipulating raw data to make it more suitable for analysis, storage, or integration with other systems.Â
At its core, data transformation ensures that data becomes more valuable and usable for business purposes.
The fundamentals of data transformation
Data transformation encompasses several key techniques and processes. These processes are often performed using data structures, like dataframes, which allow for efficient data manipulation:
- Data cleaning and preprocessing: This involves identifying and correcting errors, inconsistencies, and inaccuracies in raw data. Common tasks include removing duplicates, handling missing values, and standardizing formats (such as converting all dates to YYYY-MM-DD).
- Data normalization and standardization: This process scales numerical data to standard ranges, ensuring all variables contribute equally to analysis and modeling.
- Data encoding: Converting categorical data into numerical formats that can be processed by algorithms, using techniques like one-hot encoding or label encoding.
- Data aggregation: Combining multiple data points into summary statistics, such as calculating daily sales totals from individual transaction records.
- Data restructuring: Changing the organization or layout of data, like pivoting tables or converting between wide and long formats.
- Data testing: Ensuring transformed data meets quality standards and business rules before further processing or analysis, through both automated validation checks and manual testing procedures.
Why data transformation is critical in modern data management
Data transformation is crucial in today's data-driven business environment for several reasons:
- Data quality and consistency: Transformation processes clean and standardize data, improving overall quality. Netflix uses data transformation to clean and standardize viewing data from millions of users across different devices, enabling them to provide personalized recommendations.
- Integration of disparate sources: Modern businesses deal with data from various sources in different formats. Data transformation enables the integration of these disparate sources into a unified view.
- Scalability and performance: Properly transformed data can be more efficiently stored and processed, improving system performance.
- Advanced analytics and AI/ML: Transformed data is more suitable for advanced analytics and machine learning applications, which require data in specific formats and structures.
- Data democratization: Transformed data is often more accessible and understandable to non-technical users, promoting data democratization within organizations. As noted by industry experts, "Data transformation is the bridge between raw data and actionable insights. It empowers everyone in an organization to make data-driven decisions."
ETL vs ELT: Different approaches to data transformation
There are two primary approaches to data transformation in modern systems: ETL (Extract, Transform, Load) and ELT (Extract, Load, Transform). They differ mainly in when and where the transformation occurs.
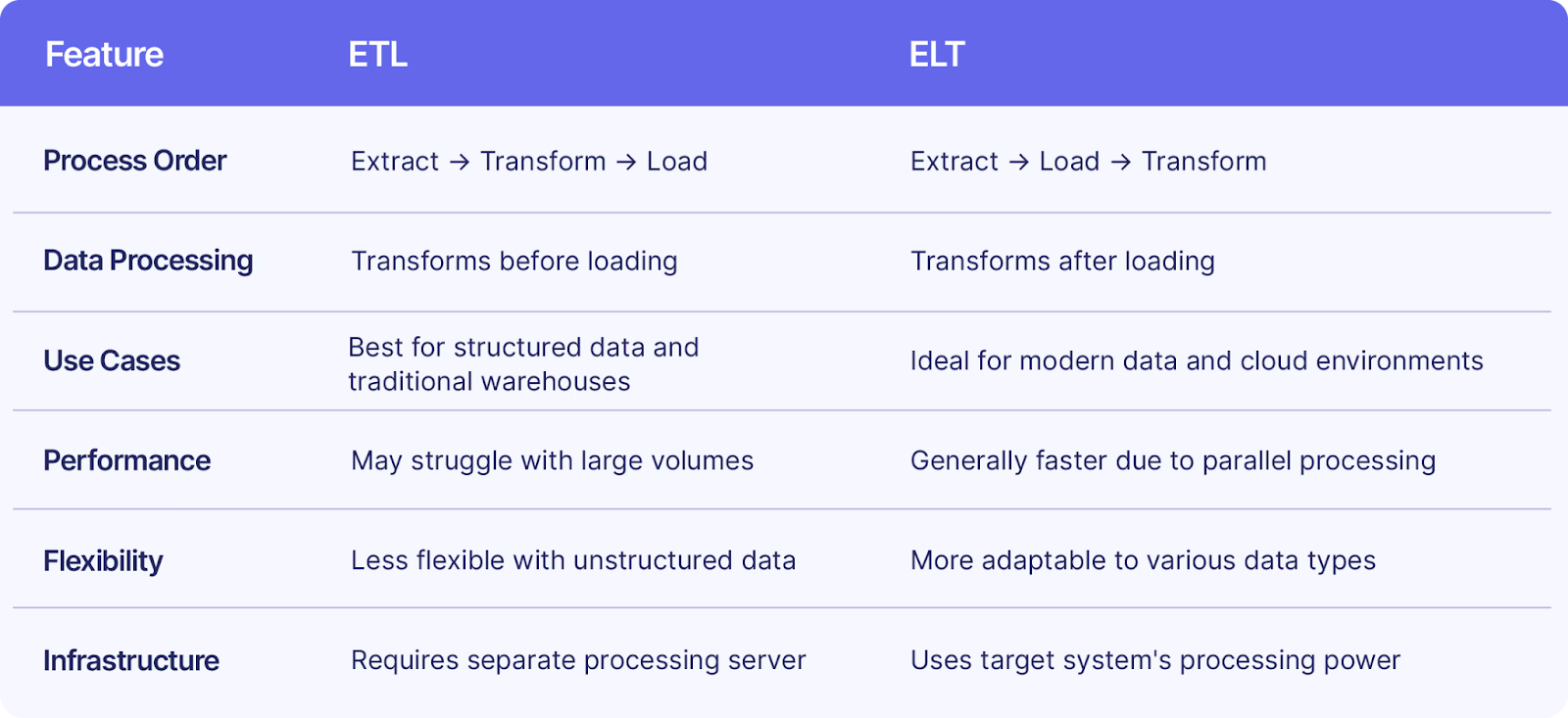
ETL process
In the ETL approach:
- Data is extracted from source systems.
- Transformed in a separate staging area.
- Loaded into the target system (typically a data warehouse).
Understanding the strengths and limitations of ETL processes is essential for designing effective data transformation strategies. ETL is better suited for structured data that fits into tables and scenarios requiring complex transformations on smaller datasets.
Environments with strict data quality and governance requirements are also ideal for ETL, as are data sources with predetermined data formats.
As organizations adapt to changing data landscapes, modern ETL practices have evolved to address new challenges in scalability and complexity.
ELT process
In the ELT approach:
- Data is extracted from source systems.
- Loaded directly into the target system.
- Transformed within the target system itself.
ELT offers several advantages:
- Faster data ingestion and real-time analytics capabilities.
- Greater flexibility and scalability, especially for big data.
- Better use of modern data warehouse processing power.
- Preservation of raw data for future analysis needs.
With ELT methodologies, organizations can leverage the processing power of modern data warehouses to handle large data volumes efficiently.Â
ELT is generally more scalable, especially for large data volumes, as it uses the computing power of the target system rather than requiring a separate transformation engine.
Types of data transformation
Let's explore the primary types of data transformation techniques that are essential in modern data management:
- Data cleaning and preprocessing: Identifies and corrects errors, inconsistencies, and inaccuracies in raw data, including removing duplicates, handling missing values, and standardizing formats.
- Data normalization and standardization: Scales numerical data to a standard range (typically between 0 and 1), ensuring all variables contribute equally to analysis and modeling.
- Data encoding: Converts categorical data into numerical formats that can be processed by algorithms, using techniques like one-hot encoding, label encoding, and binary encoding.
- Data aggregation: Combines multiple data points into summary statistics, such as calculating daily sales totals from individual transaction records.
- Data filtering: Selects a subset of data based on specific criteria, helping focus analysis on relevant information.
- Data joining/merging: Combines data from multiple sources based on common fields, creating more comprehensive datasets for analysis.
- Data type conversion: Changes the data type of variables, such as converting strings to integers or dates to ensure compatibility across systems.
- Data enrichment: Adds additional context or information to enhance the value of existing data, often by integrating third-party data sources.
- Data restructuring: Changes the organization or layout of data, such as pivoting tables or converting between wide and long formats.
- Data validation: Ensures transformed data meets quality standards and business rules before further processing or analysis.
Each type serves a specific purpose in making your data more valuable and actionable for business intelligence and analytics.
Steps in the data transformation process
Here's a step-by-step guide to effective data transformation:
1. Data identification and planning
Before diving into transformation, you need to clearly define your objectives and identify the data sources required. This involves understanding what business questions you're trying to answer and determining which data will help you get there.Â
Take time to plan your transformation workflow, identifying key dependencies and potential challenges upfront.
2. Data extraction
The next step involves pulling data from various sources—databases, cloud storage, APIs, and other repositories. Depending on your needs, this extraction can be performed as a one-time process or as an ongoing operation through scheduled jobs.Â
For complex ecosystems, you might need to integrate multiple extraction methods to ensure all relevant data is captured. Developing effective data ingestion strategies is crucial during this stage.
3. Data cleaning and assessment
Raw data often contains errors, inconsistencies, and gaps that can undermine your analysis. In this critical step, you'll need to identify and rectify issues such as missing values, duplicates, and formatting inconsistencies.Â
Data cleansing ensures that your information is accurate and reliable before proceeding with further transformations.
4. Data structuring and transformation
Now comes the core transformation stage where you convert your data into the desired format. This may involve:
- Normalizing numerical values to ensure consistency.
- Standardizing formats (dates, currencies, units).
- Encoding categorical data into numerical values.
- Aggregating individual records into summary statistics.
- Filtering out irrelevant information.
5. Data validation and testing
After transformation, it's essential to validate your data to ensure it meets quality standards and business rules. This involves checking for data integrity, consistency, and accuracy.
This step includes both automated validation tools that can flag anomalies and dedicated testing procedures that verify the transformation is working correctly. Testing should include:
- Automated data quality checks that flag anomalies or violations of business rules
- Manual validation of critical data points by domain experts
- Business-specific tests tailored to your transformation's intended outcomes
- Comparison testing between the source and transformed data to ensure accuracy
- Performance testing to verify the transformation processes at scale
Implementing both validation and testing ensures your transformed data is not only technically sound but also fit for its intended business purpose.
6. Data loading
The final step is loading your transformed data into its destination system—typically a data warehouse, data lake, or specialized analytics platform. This loading process must be carefully managed to maintain data integrity and ensure the information is readily accessible for its intended use.
Data transformation challenges and how to overcome them
Data transformation, while essential, comes with significant challenges that can impede your organization's ability to derive value from data. Understanding these obstacles and implementing strategic solutions is crucial for successful data initiatives.
1. Data quality issues
Poor data quality represents one of the most persistent challenges in data transformation. Inconsistent formats, missing values, duplicates, and inaccuracies can significantly undermine transformation efforts and lead to flawed insights.
To overcome this challenge:
- Implement robust data validation rules at the source whenever possible
- Develop comprehensive data cleaning processes with clear quality metrics
- Establish automated monitoring systems that flag quality issues early
- Document data quality standards and communicate them across the organization
2. Scalability concerns
As data volumes grow exponentially, traditional transformation approaches often struggle to scale effectively, leading to performance bottlenecks and processing delays. Our survey reveals that scalability is the leading data system challenge in modern companies, with 42% of respondents agreeing.
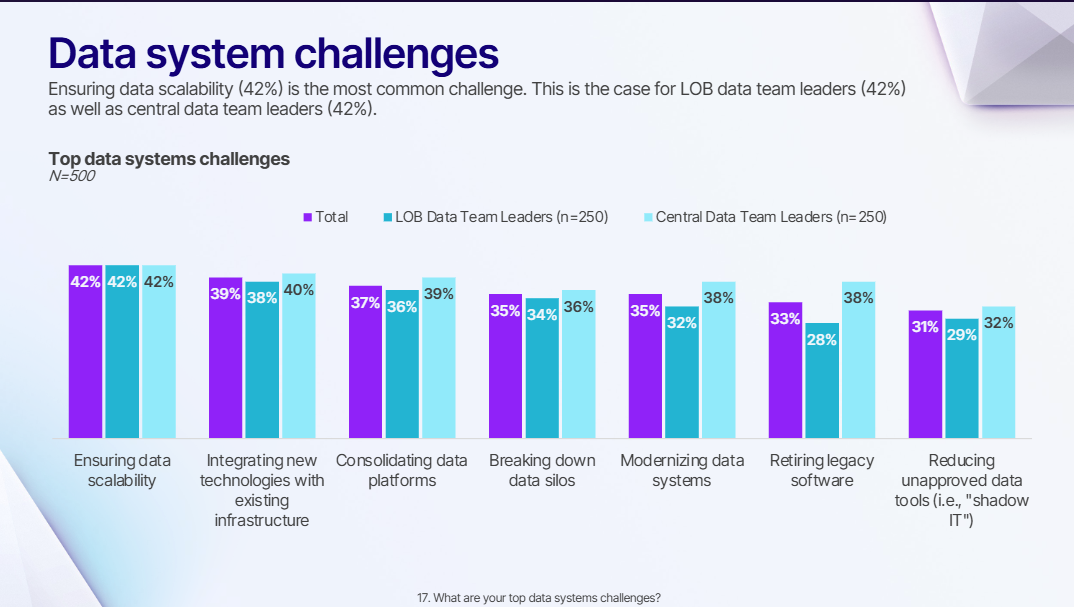
Effective solutions include:
- Adopting cloud-based transformation tools that offer elastic scaling
- Implementing parallel processing frameworks like Apache Spark
- Considering incremental processing approaches rather than full data reloads
- Optimizing transformation logic to minimize computational complexity
3. Technical complexity
Modern data ecosystems involve diverse data sources, formats, and destinations, creating significant technical complexity that requires specialized expertise.
To address this challenge:
- Invest in tools with pre-built connectors for common data sources
- Develop a clear architectural blueprint for your data transformation workflows
- Create reusable transformation components to minimize duplicated effort
- Consider low-code platforms that abstract away technical complexity
4. Governance and compliance
Regulatory requirements like GDPR, HIPAA, and industry-specific mandates create significant transformation challenges, particularly around sensitive data handling.
Effective approaches include:
- Implementing data classification to identify sensitive information
- Building transformation workflows that enforce compliance requirements
- Maintaining comprehensive data lineage documentation
- Establishing clear access controls for transformed data
5. Change management
Business requirements constantly evolve, requiring transformation processes to adapt quickly without disrupting existing data flows.
To manage this challenge:
- Adopt version control for transformation logic
- Implement CI/CD pipelines for data transformation code
- Design modular transformation workflows that can be modified independently
- Establish clear processes for testing changes before production deployment
How to choose the right data transformation tool
Selecting the appropriate data transformation tool is critical for your organization's data strategy. The right choice depends on various factors including your specific requirements, existing infrastructure, and team capabilities.
Assess your requirements
Begin by clearly defining your transformation needs:
- Data volume and complexity: Consider the size and complexity of your datasets
- Transformation types: Identify the specific transformations you'll regularly perform
- Latency requirements: Determine if you need real-time, near real-time, or batch processing
- Integration needs: List all the source and destination systems you need to connect
Document these requirements thoroughly to serve as evaluation criteria during the selection process.
Consider your technical environment
Your existing infrastructure (like Databricks) and technical ecosystem should influence your choice:
- Cloud vs. on-premises: Select tools compatible with your deployment preferences
- Programming languages: Choose solutions that align with your team's technical skills
- Existing tools: Find products that integrate well with your current data stack
- Security requirements: Ensure tools meet your organization's security standards
The ideal solution should complement rather than disrupt your established systems.
Evaluate key capabilities
Compare tools based on their core functionalities:
- Connectivity: Ensure native support for your data sources and destinations
- Scalability: Verify the tool can handle your current and future data volumes
- Usability: Look for intuitive interfaces appropriate for your team's technical level
- Performance: Check processing speeds and resource efficiency
- Debugging and monitoring: Assess the tool's capabilities for troubleshooting
Request demonstrations focused on your specific use cases rather than generic presentations.
Factor in total cost of ownership
Look beyond license fees to understand the complete financial picture:
- Implementation costs: Consider setup, integration, and migration expenses
- Operational overhead: Evaluate ongoing maintenance requirements
- Training needs: Factor in the learning curve and training expenses
- Scaling costs: Understand how expenses will change as data volumes grow
Calculate a three-year total cost of ownership to make accurate comparisons between options.
Prioritize future-proofing
Select tools that will remain viable as your needs evolve:
- Vendor stability: Research the provider's financial health and market position
- Development roadmap: Look for continuous innovation and feature expansion
- Standards compliance: Prefer tools that adhere to industry standards
- Community support: Evaluate the size and activity of the user community
The right tool should serve not only your current needs but also support your long-term data strategy.
Emerging data transformation trends
The data transformation landscape is changing, with new technologies and methodologies emerging to address the growing complexity and volume of data. Here are some of the most critical ones.
AI-powered transformation
Artificial intelligence is revolutionizing data transformation by automating complex processes and improving accuracy. AI-powered systems can learn optimal data flow routes and optimize transformation pipelines over time without human intervention.Â
These tools are particularly effective at identifying patterns, anomalies, and relationships within data that might be missed through traditional methods.
These tools use natural language processing to automate data transformations, making the process more accessible to users without extensive technical expertise. This democratization of data transformation is breaking down barriers between technical and business teams, allowing more stakeholders to participate in the data transformation process.
Shift from ETL to ELT
There's a significant shift occurring from traditional Extract, Transform, Load (ETL) processes to Extract, Load, Transform (ELT) methodologies.
This trend is being driven by several factors:
- Cloud data warehouses have become powerful enough to handle transformation workloads.
- ELT allows organizations to maintain raw data for future use cases.
- The approach is generally faster and more scalable for large datasets.
ELT processes can transform data in parallel, using the processing power of modern data warehouses, while ETL has an additional step before loading that is difficult to scale and slows down as data size increases.
Real-time data processing
The demand for real-time insights is driving the adoption of streaming data transformation technologies. Industries like finance, e-commerce, and manufacturing are increasingly implementing tools that can transform data as it arrives, rather than in scheduled batches.
This trend enables organizations to respond to events as they happen, whether it's detecting fraudulent transactions, adjusting pricing strategies, or optimizing manufacturing processes. Technologies like Apache Kafka, Apache Flink, and cloud-based streaming services are becoming essential components of modern data transformation architectures.
Visual data transformation tools
The rise of visual data transformation tools is enabling all data users to create data transformations with low-code and no-code approaches. These user-friendly interfaces allow business users to perform complex data transformations without extensive coding knowledge, accelerating time-to-insight and reducing bottlenecks.
Tools in this category offer visual interfaces for designing transformation workflows, pre-built connectors for common data sources, and templates for standard transformation patterns. This trend is particularly important as organizations face skills shortages in data engineering and analytics roles.
Cloud-native transformation solutions
Cloud-based data transformation solutions are becoming the norm rather than the exception. These platforms offer scalability, flexibility, and cost-effectiveness that on-premises solutions struggle to match.
They integrate seamlessly with their respective data storage and analytics services, creating cohesive ecosystems for end-to-end data management. This integration reduces complexity and accelerates implementation time for data transformation initiatives.
Enhanced data governance and compliance
As data privacy regulations like GDPR, CCPA, and industry-specific mandates continue to evolve, data transformation processes are increasingly incorporating governance and compliance capabilities. Investing in training and establishing data governance frameworks is becoming essential for organizations.
Modern transformation tools now include features for data lineage tracking, sensitive data identification, access controls, and audit trails. These capabilities ensure that data transformation processes not only deliver business value but also maintain regulatory compliance and data security.
DataOps and agile methodologies
Organizations are increasingly adopting DataOps principles and agile methodologies for their data transformation processes. This approach emphasizes collaboration between data engineers, data scientists, and business users while focusing on automation, testing, and continuous improvement.
By implementing DataOps practices, organizations can reduce the time required to implement data transformation workflows, improve quality through automated testing, and respond more quickly to changing business requirements.
The future of data transformation lies in embracing these emerging trends while maintaining focus on delivering business value through high-quality, accessible data. Organizations that adopt these technologies and methodologies will be better positioned to use their data assets for competitive advantage.
Examples of successful data transformation
When done right, data transformation creates a self-service environment where business users can participate in the analytics process while maintaining governance and quality. Let's look at three organizations that have successfully transformed their data operations.
CZ
CZ, a healthcare insurance company with over 3,000 employees, faced significant challenges with their legacy data infrastructure. The company was struggling with siloed systems—including SAS and Cloudera—that created bottlenecks where limited engineering expertise led to delays in data access and hampered business agility.
Their transformation journey involved migrating from these disparate systems to a modern Databricks platform with Prophecy's visual data transformation tools. This strategic shift yielded impressive results:
- The company migrated over 2,000 workflows to Databricks.
- Prophecy has facilitated self-service analytics for business users across various units.
- Significantly reduced dependency on the central data team.
By democratizing pipeline development with AI-powered visual design tools, CZ empowered business analysts to validate and develop their own data pipelines.Â
This acceleration of insights fostered a data mesh architecture that improved their ability to deliver healthcare insurance services to their customers.
Aetion
Aetion, a healthcare and life sciences company with over 200 employees, successfully launched their real-world evidence platform "Discover" by implementing advanced data transformation tools. The company was struggling with legacy data architecture and manual processes that created bottlenecks in data operations and delayed product launches.
Aetion adopted Prophecy's visual, no-code interface with drag-and-drop functionality and reusable components to enhance their workflow. The results were transformative:
- The company processed 500 million health records in two months.
- Data source onboarding time has increased by 50%.
- Streamlined development workflows and eliminated manual work.
This transformation accelerated pipeline creation, allowing Aetion to not only meet their Discover product launch deadline but also onboard their first three customers ahead of schedule while providing faster insights for healthcare outcomes.
Waterfall Asset Management
Waterfall Asset Management, an $11.2 billion financial services firm, dramatically improved their investment decision-making process by implementing a low-code data engineering platform on the Databricks Lakehouse.Â
Previously hampered by manual processes and a legacy ETL system that couldn't handle their data scale, the company transformed their operations by democratizing data access and empowering business users with intuitive, self-service tools.
The transformation produced remarkable results:
- Data engineering workflows become 14x more efficient, reducing the time from weeks to just half a day.
- Trade desk analysts receive data 4x faster than before.
The platform's ability to automatically generate 100% open-source Spark code enabled engineers to maintain best practices while allowing business users to create data pipelines without coding knowledge.
Better data transformation for better insights
Data transformation has evolved significantly over the years, moving from simple ETL processes to more sophisticated approaches that use cloud computing, AI, and automation. Prophecy helps organizations navigate this changing landscape by providing powerful capabilities that streamline and enhance data transformation processes.
Prophecy enhances data transformation with user-friendly interfaces, integration capabilities, and tools for managing data efficiently.
Learn more about AI-powered data transformation and how self-service tooling raises productivity in modern organizations.
Ready to give Prophecy a try?
You can create a free account and get full access to all features for 21 days. No credit card needed. Want more of a guided experience? Request a demo and we’ll walk you through how Prophecy can empower your entire data team with low-code ETL today.
Ready to see Prophecy in action?
Request a demo and we’ll walk you through how Prophecy’s AI-powered visual data pipelines and high-quality open source code empowers everyone to speed data transformation
Get started with the Low-code Data Transformation Platform
Meet with us at Gartner Data & Analytics Summit in Orlando March 11-13th. Schedule a live 1:1 demo at booth #600 with our team of low-code experts. Request a demo here.